AWS Lambda for Real-world Scenarios
AWS Lambda is a powerful and versatile service that allows developers to execute code without the need for provisioning and managing servers. It’s a serverless computing service that allows you to run code in response to events and automatically manages the underlying compute resources for you. In this blog, we’ll explore the basics of AWS Lambda, its features, and real-world scenarios where Lambda can be used to build scalable and efficient applications.
What is AWS Lambda?
AWS Lambda is an event-driven computing service that allows you to run code in response to events like changes to data in an Amazon S3 bucket, or a new record in an Amazon DynamoDB table. With AWS Lambda, you can write your code in a variety of programming languages, including Node.js, Python, Java, C#, and Go. You can also integrate your Lambda functions with other AWS services, such as Amazon S3, Amazon DynamoDB, and Amazon API Gateway.
The key benefits of using AWS Lambda include:
- Serverless: You don’t need to manage servers, operating systems, or infrastructure.
- Pay-per-use: You only pay for the compute time you consume.
- Highly scalable: AWS Lambda automatically scales your application in response to incoming traffic.
- Flexible: You can use a variety of programming languages and integrate with other AWS services.
Real-world Scenarios
Let’s explore a few real-world scenarios where AWS Lambda can be used:
1. Image Processing
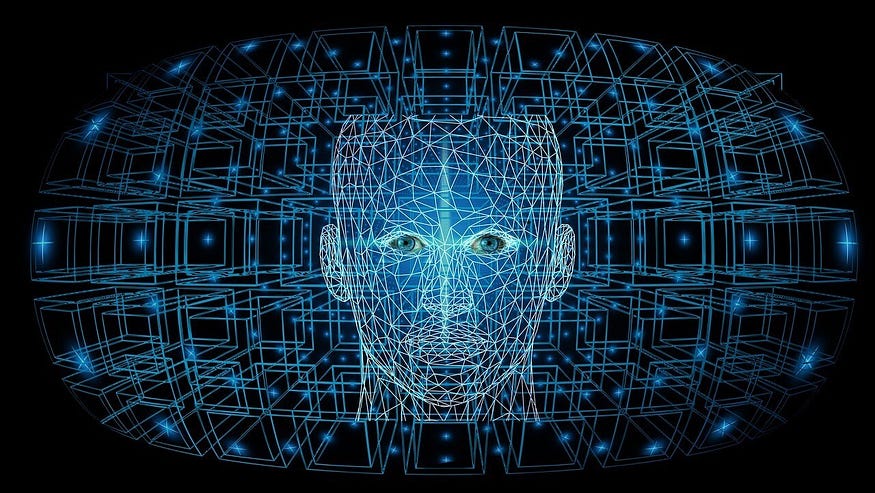
Image processing is a common use case for AWS Lambda. For example, you might have an application that allows users to upload images, and you need to perform some processing on those images, such as resizing, cropping, or adding watermarks. You can use Lambda to process the images in response to an S3 event trigger. Here’s an example image processing Lambda function in Python using Pillow, a popular Python library for image processing:
import boto3
from io import BytesIO
from PIL import Image
s3 = boto3.client('s3')
def lambda_handler(event, context):
# Get the bucket and object key from the event
bucket = event['Records'][0]['s3']['bucket']['name']
key = event['Records'][0]['s3']['object']['key']
# Read the image file from S3
response = s3.get_object(Bucket=bucket, Key=key)
image_binary = response['Body'].read()
# Open the image using Pillow
image = Image.open(BytesIO(image_binary))
# Resize the image to a smaller size
resized_image = image.resize((int(image.width / 2), int(image.height / 2)))
# Convert the image to JPEG format and save it to S3
output_buffer = BytesIO()
resized_image.save(output_buffer, format='JPEG')
s3.put_object(Body=output_buffer.getvalue(), Bucket=bucket, Key='resized/' + key)
return {
'statusCode': 200,
'body': 'Image processed successfully'
}
This code uses the AWS SDK for Python (Boto3) to read an image file from an S3 bucket, opens it using Pillow, resizes the image to a smaller size, converts it to JPEG format, and saves the resized image back to the same S3 bucket under a different prefix. The function is triggered by an S3 event, which is sent to the function whenever a new file is uploaded to the source S3 bucket. Note that this code assumes that the input image file is in JPEG format, but you can modify the code to handle other image formats as well.
This code resizes images to 500x500 pixels and saves the resized images to a “resized” folder in the same S3 bucket.
2. Chatbots
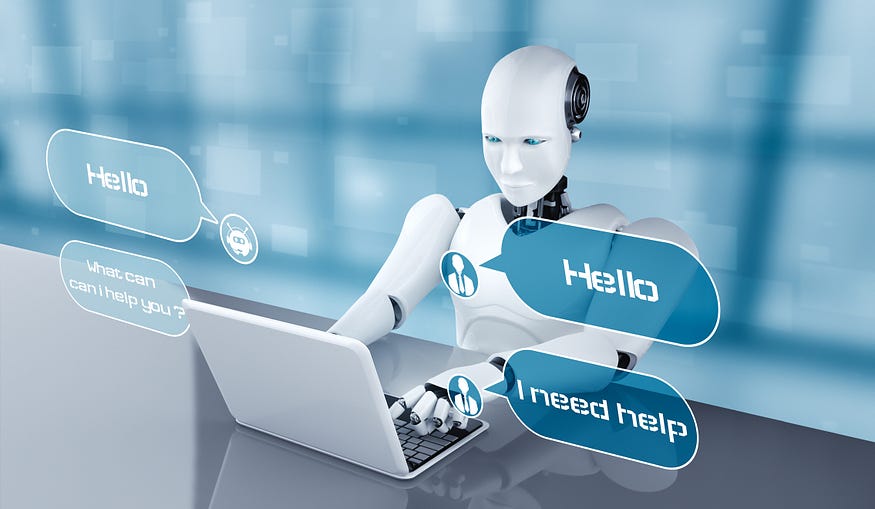
Chatbots are another popular use case for AWS Lambda. You can use Lambda to build conversational interfaces for your applications, such as chatbots for customer support, or voice assistants for smart home devices. You can integrate your Lambda function with Amazon Lex, a service that allows you to build chatbots with natural language understanding. Here’s an example Python code snippet for a Lambda function that responds to user input with a greeting:
import json
def lambda_handler(event, context):
user_input = event['currentIntent']['slots']['UserInput']
if user_input == 'hello':
response = {
"dialogAction": {
"type": "Close",
"fulfillmentState": "Fulfilled",
"message": {
"contentType": "PlainText",
"content": "Hi there!"
}
}
}
else:
response = {
"dialogAction": {
"type": "ElicitIntent",
"message": {
"contentType": "PlainText",
"content": "I didn't understand. Can you please say that again?"
}
}
}
return response
The Lambda function is triggered by an event from Amazon Lex, which sends a user input string to the function. The function then checks the user input and responds with a greeting if the input is “hello”, or asks the user to repeat the input if it’s not understood.
3. Data Processing
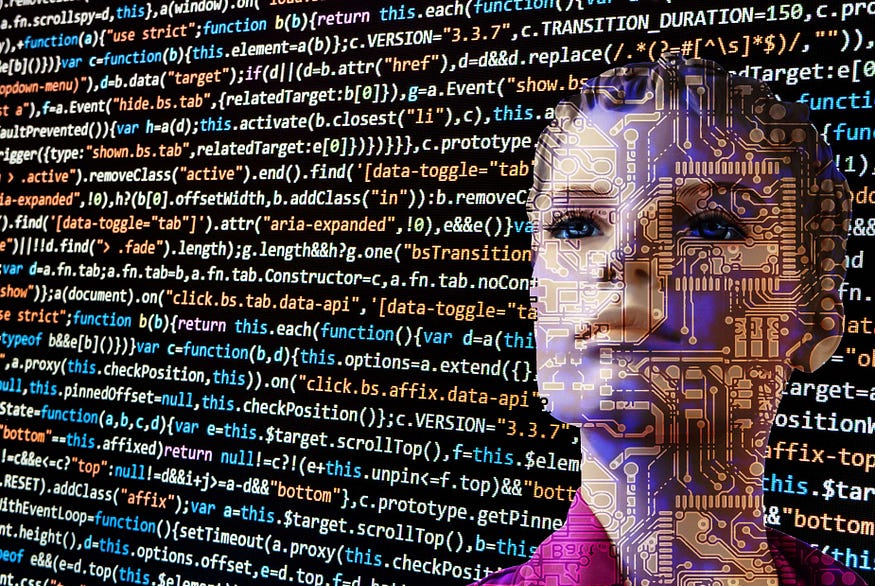
Data processing is another common use case for AWS Lambda. You can use Lambda to process data in real-time, such as streaming data from Amazon Kinesis, or processing batch data from Amazon S3. For example, you might have a large amount of data stored in an S3 bucket, and you need to process that data in parallel to save time. You can use Lambda to process the data in parallel, and write the results to another S3 bucket. Here’s an example Python code snippet that processes CSV data from S3:
import boto3
import pandas as pd
s3 = boto3.client('s3')
def lambda_handler(event, context):
bucket = event['Records'][0]['s3']['bucket']['name']
key = event['Records'][0]['s3']['object']['key']
obj = s3.get_object(Bucket=bucket, Key=key)
data = pd.read_csv(obj['Body'])
# Perform some data processing
processed_data = data.dropna()
# Write the processed data to a new S3 bucket
s3.put_object(Body=processed_data.to_csv(index=False), Bucket='processed-bucket', Key=key)
return {
'statusCode': 200,
'body': 'Data processed successfully'
}
This code reads a CSV file from an S3 bucket, performs some data processing (in this case, dropping any rows with missing values), and writes the processed data to a new S3 bucket. The function is triggered by an S3 event, which is sent to the function whenever a new file is uploaded to the source S3 bucket.
Conclusion
AWS Lambda is a powerful serverless computing service that allows you to run code in response to events. You can use Lambda to build scalable and efficient applications for a variety of use cases, including image processing, chatbots, and data processing. With Lambda, you don’t need to worry about managing servers or infrastructure, and you only pay for the compute time you consume. I hope this blog has given you a good introduction to AWS Lambda and some ideas for how you can use it in your own applications.
If this post was helpful, please do follow and click the clap 👏 button below to show your support 😄
_ Thank you for reading💚
Follow me on LinkedIn💙
A Comprehensive Guide to Amazon Web Services (AWS) Services💻
AWS, or Amazon Web Services, is a collection of digital infrastructure services that can be used to build and run a wide variety of applications and services. These services can be divided into several broad categories, including compute, storage, databases, and network and content delivery. In this article, we will take a detailed look at each of these categories and the individual services that make them up.
Compute Services
- EC2 (Elastic Compute Cloud): This is the core compute service in AWS, and allows you to launch virtual machines (VMs) in the cloud. VMs can be launched in a variety of configurations, including different operating systems, CPU and memory sizes, and network configurations.
- Elastic Beanstalk: This service is a higher-level service that sits on top of EC2, and allows you to deploy and run web applications with minimal setup and management.
- Lambda: This service allows you to run code in the cloud without the need to provision or manage servers. You can write code in a variety of languages, and AWS will automatically run and scale the code as needed.
- Batch: This service allows you to run batch jobs in the cloud, such as processing large data sets or running long-running computations.
Storage Services
- S3 (Simple Storage Service): This is the core storage service in AWS, and allows you to store and retrieve large amounts of data in the cloud. S3 is object-based storage, which means that you can store individual files or “objects” in the service, and access them via a unique URL.
- EBS (Elastic Block Store): This service provides block-level storage for use with EC2 instances. This can be used for applications that require a higher level of IOPS performance
- Glacier: This service is a low-cost storage service that is well-suited for archival data.
- Storage Gateway: This service allows you to store data on-premises and in the cloud, and provides a bridge between the two.
Container Services
- ECS (Elastic Container Service): This service allows you to run and manage Docker containers on a cluster of EC2 instances. ECS provides native support for Docker images and can be used to deploy and scale containerized applications.
- ECR (Elastic Container Registry): This service allows you to store and manage your own Docker images in the cloud. ECR is fully integrated with ECS and can be used to store, manage, and deploy container images.
- Fargate: This service allows you to run containers in the cloud without the need to manage the underlying infrastructure. You can simply specify the number of containers that you want to run and AWS will take care of the rest.
AWS also provides a Kubernetes service called EKS (Elastic Kubernetes Service) which makes it easy to run, scale and manage containerized applications using Kubernetes on AWS. Additionally, AWS App Runner and CodeBuild can be used for CI/CD for containerized applications.
Database Services
- RDS (Relational Database Service): This service allows you to run a variety of relational databases in the cloud, including MySQL, PostgreSQL, and Oracle.
- DynamoDB: This service is a NoSQL database service that provides a fast and flexible way to store and retrieve data.
- Redshift: This service is a data warehousing service that allows you to run complex queries on large amounts of data.
- Neptune: This service is a graph database service that makes it easy to store and query data that is connected by relationships
Network and Content Delivery Services
- VPC (Virtual Private Cloud): This service allows you to create a virtual network in the cloud, complete with its own IP address range, subnets, and routing tables.
- CloudFront: This service is a content delivery network (CDN) that allows you to distribute content to users around the world with low latency and high transfer speeds.
- Route 53: This service is a domain name system (DNS) service that allows you to map domain names to IP addresses, and manage your DNS records.
- Direct Connect: This service allows you to establish a dedicated connection between your on-premises infrastructure and the AWS cloud.
Additional Services:
- IAM (Identity and Access Management): This service allows you to control access to AWS resources and services. You can use IAM to create and manage users, groups, and permissions, and to enforce least privilege access policies.
- CloudFormation: This service allows you to use templates to provision and manage resources across multiple services in your AWS infrastructure.
- CloudWatch: This service allows you to monitor your AWS resources and applications in real-time, and to set alarms and take automated actions in response to changes in performance or availability.
- CloudTrail: This service allows you to log and monitor all AWS API calls made in your AWS account, including calls made by the AWS Management Console, SDKs, command line tools, and higher-level services like CloudFormation.
- KMS (Key Management Service): This service allows you to create and manage encryption keys that you can use to encrypt data stored in AWS services.
AWS also provides a wide range of other services such as Elasticsearch, SQS, SNS, SES, AppSync, AppMesh, ElasticTranscoder, Elasticsearch Service, Elasticsearch for Kubernetes, QuickSight, Pinpoint, Personalize, SageMaker, Comprehend, Transcribe, Translate, and many more. Each of these services can be used to build and run various types of applications and services, and can be combined and integrated with other services to create a complete solution.
In conclusion, AWS provides a vast array of services that can be used to build and run a wide variety of applications and services. By understanding the different categories of services and the individual services that make them up, you can choose the right services for your specific needs and build a solution that is tailored to your requirements.
6 Strategies for Migrating Applications to the Cloud
Formulating a Migration Strategy
Enterprises typically begin to contemplate how to migrate an application during the second phase of the “Migration Process” — Portfolio Discovery and Planning. This is when they determine what’s in their environment, what are the interdependencies, what’s going to be easy to migrate and what’s going to be hard to migrate, and how they’ll migrate each application.
Using this knowledge, organizations can outline a plan (which should be considered subject to change as they progress through their migration and learn) on how they’ll approach migrating each of the applications in their portfolio and in what order.
The complexity of migrating existing applications varies, depending on the architecture and existing licensing arrangements. If I think about the universe of applications to migrate on a spectrum of complexity, I’d put a virtualized, service-oriented architecture on the low-complexity end of the spectrum, and a monolithic mainframe at the high-complexity end of the spectrum.
I suggest starting with something on the low-complexity end of the spectrum for the obvious reason that it will be easier to complete — which will give you some immediate positive reinforcement or “quick wins” as you learn.
6 Application Migration Strategies: “The 6 R’s”
The 6 most common application migration strategies we see are:1. Rehosting — Otherwise known as “lift-and-shift.”
We find that many early cloud projects gravitate toward net new development using cloud-native capabilities, but in a large legacy migration scenario where the organization is looking to scale its migration quickly to meet a business case, we find that the majority of applications are rehosted. GE Oil & Gas, for instance, found that, even without implementing any cloud optimizations, it could save roughly 30 percent of its costs by rehosting.
Most rehosting can be automated with tools (e.g. AWS VM Import/Export, Racemi), although some customers prefer to do this manually as they learn how to apply their legacy systems to the new cloud platform.
We’ve also found that applications are easier to optimize/re-architect once they’re already running in the cloud. Partly because your organization will have developed better skills to do so, and partly because the hard part — migrating the application, data, and traffic — has already been done.
2. Replatforming — I sometimes call this “lift-tinker-and-shift.”
Here you might make a few cloud (or other) optimizations in order to achieve some tangible benefit, but you aren’t otherwise changing the core architecture of the application. You may be looking to reduce the amount of time you spend managing database instances by migrating to a database-as-a-service platform like Amazon Relational Database Service (Amazon RDS), or migrating your application to a fully managed platform like Amazon Elastic Beanstalk.
A large media company we work with migrated hundreds of web servers it ran on-premises to AWS, and, in the process, it moved from WebLogic (a Java application container that requires an expensive license) to Apache Tomcat, an open-source equivalent. This media company saved millions in licensing costs on top of the savings and agility it gained by migrating to AWS.
3. Repurchasing — Moving to a different product.
I most commonly see repurchasing as a move to a SaaS platform. Moving a CRM to Salesforce.com, an HR system to Workday, a CMS to Drupal, and so on.4. Refactoring / Re-architecting — Re-imagining how the application is architected and developed, typically using cloud-native features.
This is typically driven by a strong business need to add features, scale, or performance that would otherwise be difficult to achieve in the application’s existing environment.Are you looking to migrate from a monolithic architecture to a service-oriented (or server-less) architecture to boost agility or improve business continuity (I’ve heard stories of mainframe fan belts being ordered on e-bay)? This pattern tends to be the most expensive, but, if you have a good product-market fit, it can also be the most beneficial.